Data Analysis - 7 Angebote vergleichen
Bester Preis: € 133,99 (vom 06.06.2016)1

Co-Clustering

ISBN: 9781118649497 bzw. 1118649494, in Englisch, Wiley, neu, E-Book, elektronischer Download.
Lieferung aus: Vereinigtes Königreich Großbritannien und Nordirland, Despatched same working day before 3pm.
Cluster or co-cluster analyses are important tools in a variety of scientific areas. The introduction of this book presents a state of the art of already well-established, as well as more recent methods of co-clustering. The authors mainly deal with the two-mode partitioning under different approaches, but pay particular attention to a probabilistic approach. Chapter 1 concerns clustering in general and the model-based clustering in particular. The authors briefly review the classical clustering methods and focus on the mixture model. They present and discuss the use of different mixtures adapted to different types of data. The algorithms used are described and related works with different classical methods are presented and commented upon. This chapter is useful in tackling the problem of co-clustering under the mixture approach. Chapter 2 is devoted to the latent block model proposed in the mixture approach context. The authors discuss this model in detail and present its interest regarding co-clustering. Various algorithms are presented in a general context. Chapter 3 focuses on binary and categorical data. It presents, in detail, the appropriated latent block mixture models. Variants of these models and algorithms are presented and illustrated using examples. Chapter 4 focuses on contingency data. Mutual information, phi-squared and model-based co-clustering are studied. Models, algorithms and connections among different approaches are described and illustrated. Chapter 5 presents the case of continuous data. In the same way, the different approaches used in the previous chapters are extended to this situation.Contents1. Cluster Analysis. 2. Model-Based Co-Clustering. 3. Co-Clustering of Binary and Categorical Data. 4. Co-Clustering of Contingency Tables. 5. Co-Clustering of Continuous Data.About the AuthorsG rard Govaert is Professor at the University of Technology of Compi gne, France. He is also a member of the CNRS Laboratory Heudiasyc (Heuristic and diagnostic of complex systems). His research interests include latent structure modeling, model selection, model-based cluster analysis, block clustering and statistical pattern recognition. He is one of the authors of the MIXMOD (MIXtureMODelling) software. Mohamed Nadif is Professor at the University of Paris-Descartes, France, where he is a member of LIPADE (Paris Descartes computer science laboratory) in the Mathematics and Computer Science department. His research interests include machine learning, data mining, model-based cluster analysis, co-clustering, factorization and data analysis.Cluster Analysis is an important tool in a variety of scientific areas. Chapter 1 briefly presents a state of the art of already well-established as well more recent methods. The hierarchical, partitioning and fuzzy approaches will be discussed amongst others. The authors review the difficulty of these classical methods in tackling the high dimensionality, sparsity and scalability. Chapter 2 discusses the interests of coclustering, presenting different approaches and defining a co-cluster. The authors focus on co-clustering as a simultaneous clustering and discuss the cases of binary, continuous and co-occurrence data. The criteria and algorithms are described and illustrated on simulated and real data. Chapter 3 considers co-clustering as a model-based co-clustering. A latent block model is defined for different kinds of data. The estimation of parameters and co-clustering is tackled under two approaches: maximum likelihood and classification maximum likelihood. Hard and soft algorithms are described and applied on simulated and real data. Chapter 4 considers co-clustering as a matrix approximation. The trifactorization approach is considered and algorithms based on update rules are described. Links with numerical and probabi.
Cluster or co-cluster analyses are important tools in a variety of scientific areas. The introduction of this book presents a state of the art of already well-established, as well as more recent methods of co-clustering. The authors mainly deal with the two-mode partitioning under different approaches, but pay particular attention to a probabilistic approach. Chapter 1 concerns clustering in general and the model-based clustering in particular. The authors briefly review the classical clustering methods and focus on the mixture model. They present and discuss the use of different mixtures adapted to different types of data. The algorithms used are described and related works with different classical methods are presented and commented upon. This chapter is useful in tackling the problem of co-clustering under the mixture approach. Chapter 2 is devoted to the latent block model proposed in the mixture approach context. The authors discuss this model in detail and present its interest regarding co-clustering. Various algorithms are presented in a general context. Chapter 3 focuses on binary and categorical data. It presents, in detail, the appropriated latent block mixture models. Variants of these models and algorithms are presented and illustrated using examples. Chapter 4 focuses on contingency data. Mutual information, phi-squared and model-based co-clustering are studied. Models, algorithms and connections among different approaches are described and illustrated. Chapter 5 presents the case of continuous data. In the same way, the different approaches used in the previous chapters are extended to this situation.Contents1. Cluster Analysis. 2. Model-Based Co-Clustering. 3. Co-Clustering of Binary and Categorical Data. 4. Co-Clustering of Contingency Tables. 5. Co-Clustering of Continuous Data.About the AuthorsG rard Govaert is Professor at the University of Technology of Compi gne, France. He is also a member of the CNRS Laboratory Heudiasyc (Heuristic and diagnostic of complex systems). His research interests include latent structure modeling, model selection, model-based cluster analysis, block clustering and statistical pattern recognition. He is one of the authors of the MIXMOD (MIXtureMODelling) software. Mohamed Nadif is Professor at the University of Paris-Descartes, France, where he is a member of LIPADE (Paris Descartes computer science laboratory) in the Mathematics and Computer Science department. His research interests include machine learning, data mining, model-based cluster analysis, co-clustering, factorization and data analysis.Cluster Analysis is an important tool in a variety of scientific areas. Chapter 1 briefly presents a state of the art of already well-established as well more recent methods. The hierarchical, partitioning and fuzzy approaches will be discussed amongst others. The authors review the difficulty of these classical methods in tackling the high dimensionality, sparsity and scalability. Chapter 2 discusses the interests of coclustering, presenting different approaches and defining a co-cluster. The authors focus on co-clustering as a simultaneous clustering and discuss the cases of binary, continuous and co-occurrence data. The criteria and algorithms are described and illustrated on simulated and real data. Chapter 3 considers co-clustering as a model-based co-clustering. A latent block model is defined for different kinds of data. The estimation of parameters and co-clustering is tackled under two approaches: maximum likelihood and classification maximum likelihood. Hard and soft algorithms are described and applied on simulated and real data. Chapter 4 considers co-clustering as a matrix approximation. The trifactorization approach is considered and algorithms based on update rules are described. Links with numerical and probabi.
2

Co-Clustering. Models, Algorithms and Applications

ISBN: 9781118649497 bzw. 1118649494, vermutlich in Englisch, John Wiley & Sons Limited, neu, E-Book, elektronischer Download.
Lieferung aus: Russische Föderation, zzgl. Versandkosten.
Cluster or co-cluster analyses are important tools in a variety of scientific areas. The introduction of this book presents a state of the art of already well-established, as well as more recent methods of co-clustering. The authors mainly deal with the two-mode partitioning under different approaches, but pay particular attention to a probabilistic approach. Chapter 1 concerns clustering in general and the model-based clustering in particular. The authors briefly review the classical clustering methods and focus on the mixture model. They present and discuss the use of different mixtures adapted to different types of data. The algorithms used are described and related works with different classical methods are presented and commented upon. This chapter is useful in tackling the problem of co-clustering under the mixture approach. Chapter 2 is devoted to the latent block model proposed in the mixture approach context. The authors discuss this model in detail and present its interest regarding co-clustering. Various algorithms are presented in a general context. Chapter 3 focuses on binary and categorical data. It presents, in detail, the appropriated latent block mixture models. Variants of these models and algorithms are presented and illustrated using examples. Chapter 4 focuses on contingency data. Mutual information, phi-squared and model-based co-clustering are studied. Models, algorithms and connections among different approaches are described and illustrated. Chapter 5 presents the case of continuous data. In the same way, the different approaches used in the previous chapters are extended to this situation. Contents 1. Cluster Analysis. 2. Model-Based Co-Clustering. 3. Co-Clustering of Binary and Categorical Data. 4. Co-Clustering of Contingency Tables. 5. Co-Clustering of Continuous Data. About the Authors Gérard Govaert is Professor at the University of Technology of Compiègne, France. He is also a member of the CNRS Laboratory Heudiasyc (Heuristic and diagnostic of complex systems). His research interests include latent structure modeling, model selection, model-based cluster analysis, block clustering and statistical pattern recognition. He is one of the authors of the MIXMOD (MIXtureMODelling) software. Mohamed Nadif is Professor at the University of Paris-Descartes, France, where he is a member of LIPADE (Paris Descartes computer science laboratory) in the Mathematics and Computer Science department. His research interests include machine learning, data mining, model-based cluster analysis, co-clustering, factorization and data analysis. Cluster Analysis is an important tool in a variety of scientific areas. Chapter 1 briefly presents a state of the art of already well-established as well more recent methods. The hierarchical, partitioning and fuzzy approaches will be discussed amongst others. The authors review the difficulty of these classical methods in tackling the high dimensionality, sparsity and scalability. Chapter 2 discusses the interests of coclustering, presenting different approaches and defining a co-cluster. The authors focus on co-clustering as a simultaneous clustering and discuss the cases of binary, continuous and co-occurrence data. The criteria and algorithms are described and illustrated on simulated and real data. Chapter 3 considers co-clustering as a model-based co-clustering. A latent block model is defined for different kinds of data. The estimation of parameters and co-clustering is tackled under two approaches: maximum likelihood and classification maximum likelihood. Hard and soft algorithms are described and applied on simulated and real data. Chapter 4 considers co-clustering as a matrix approximation. The trifactorization approach is considered and algorithms based on update rules are described. Links with numerical and probabi, Компьютеры/Программирование, book, PDF.
Cluster or co-cluster analyses are important tools in a variety of scientific areas. The introduction of this book presents a state of the art of already well-established, as well as more recent methods of co-clustering. The authors mainly deal with the two-mode partitioning under different approaches, but pay particular attention to a probabilistic approach. Chapter 1 concerns clustering in general and the model-based clustering in particular. The authors briefly review the classical clustering methods and focus on the mixture model. They present and discuss the use of different mixtures adapted to different types of data. The algorithms used are described and related works with different classical methods are presented and commented upon. This chapter is useful in tackling the problem of co-clustering under the mixture approach. Chapter 2 is devoted to the latent block model proposed in the mixture approach context. The authors discuss this model in detail and present its interest regarding co-clustering. Various algorithms are presented in a general context. Chapter 3 focuses on binary and categorical data. It presents, in detail, the appropriated latent block mixture models. Variants of these models and algorithms are presented and illustrated using examples. Chapter 4 focuses on contingency data. Mutual information, phi-squared and model-based co-clustering are studied. Models, algorithms and connections among different approaches are described and illustrated. Chapter 5 presents the case of continuous data. In the same way, the different approaches used in the previous chapters are extended to this situation. Contents 1. Cluster Analysis. 2. Model-Based Co-Clustering. 3. Co-Clustering of Binary and Categorical Data. 4. Co-Clustering of Contingency Tables. 5. Co-Clustering of Continuous Data. About the Authors Gérard Govaert is Professor at the University of Technology of Compiègne, France. He is also a member of the CNRS Laboratory Heudiasyc (Heuristic and diagnostic of complex systems). His research interests include latent structure modeling, model selection, model-based cluster analysis, block clustering and statistical pattern recognition. He is one of the authors of the MIXMOD (MIXtureMODelling) software. Mohamed Nadif is Professor at the University of Paris-Descartes, France, where he is a member of LIPADE (Paris Descartes computer science laboratory) in the Mathematics and Computer Science department. His research interests include machine learning, data mining, model-based cluster analysis, co-clustering, factorization and data analysis. Cluster Analysis is an important tool in a variety of scientific areas. Chapter 1 briefly presents a state of the art of already well-established as well more recent methods. The hierarchical, partitioning and fuzzy approaches will be discussed amongst others. The authors review the difficulty of these classical methods in tackling the high dimensionality, sparsity and scalability. Chapter 2 discusses the interests of coclustering, presenting different approaches and defining a co-cluster. The authors focus on co-clustering as a simultaneous clustering and discuss the cases of binary, continuous and co-occurrence data. The criteria and algorithms are described and illustrated on simulated and real data. Chapter 3 considers co-clustering as a model-based co-clustering. A latent block model is defined for different kinds of data. The estimation of parameters and co-clustering is tackled under two approaches: maximum likelihood and classification maximum likelihood. Hard and soft algorithms are described and applied on simulated and real data. Chapter 4 considers co-clustering as a matrix approximation. The trifactorization approach is considered and algorithms based on update rules are described. Links with numerical and probabi, Компьютеры/Программирование, book, PDF.
3

Co-Clustering - Models, Algorithms and Applications

ISBN: 9781118649497 bzw. 1118649494, in Englisch, John Wiley & Sons, neu, E-Book, elektronischer Download.
Lieferung aus: Deutschland, Free shipping.
Co-Clustering: Cluster or co-cluster analyses are important tools in a variety of scientific areas. The introduction of this book presents a state of the art of already well-established, as well as more recent methods of co-clustering. The authors mainly deal with the two-mode partitioning under different approaches, but pay particular attention to a probabilistic approach. Chapter 1 concerns clustering in general and the model-based clustering in particular. The authors briefly review the classical clustering methods and focus on the mixture model. They present and discuss the use of different mixtures adapted to different types of data. The algorithms used are described and related works with different classical methods are presented and commented upon. This chapter is useful in tackling the problem of co-clustering under the mixture approach. Chapter 2 is devoted to the latent block model proposed in the mixture approach context. The authors discuss this model in detail and present its interest regarding co-clustering. Various algorithms are presented in a general context. Chapter 3 focuses on binary and categorical data. It presents, in detail, the appropriated latent block mixture models. Variants of these models and algorithms are presented and illustrated using examples. Chapter 4 focuses on contingency data. Mutual information, phi-squared and model-based co-clustering are studied. Models, algorithms and connections among different approaches are described and illustrated. Chapter 5 presents the case of continuous data. In the same way, the different approaches used in the previous chapters are extended to this situation. Contents 1. Cluster Analysis. 2. Model-Based Co-Clustering. 3. Co-Clustering of Binary and Categorical Data. 4. Co-Clustering of Contingency Tables. 5. Co-Clustering of Continuous Data. About the Authors Gérard Govaert is Professor at the University of Technology of Compiègne, France. He is also a member of the CNRS Laboratory Heudiasyc (Heuristic and diagnostic of complex systems). His research interests include latent structure modeling, model selection, model-based cluster analysis, block clustering and statistical pattern recognition. He is one of the authors of the MIXMOD (MIXtureMODelling) software. Mohamed Nadif is Professor at the University of Paris-Descartes, France, where he is a member of LIPADE (Paris Descartes computer science laboratory) in the Mathematics and Computer Science department. His research interests include machine learning, data mining, model-based cluster analysis, co-clustering, factorization and data analysis. Cluster Analysis is an important tool in a variety of scientific areas. Chapter 1 briefly presents a state of the art of already well-established as well more recent methods. The hierarchical, partitioning and fuzzy approaches will be discussed amongst others. The authors review the difficulty of these classical methods in tackling the high dimensionality, sparsity and scalability. Chapter 2 discusses the interests of coclustering, presenting different approaches and defining a co-cluster. The authors focus on co-clustering as a simultaneous clustering and discuss the cases of binary, continuous and co-occurrence data. The criteria and algorithms are described and illustrated on simulated and real data. Chapter 3 considers co-clustering as a model-based co-clustering. A latent block model is defined for different kinds of data. The estimation of parameters and co-clustering is tackled under two approaches: maximum likelihood and classification maximum likelihood. Hard and soft algorithms are described and applied on simulated and real data. Chapter 4 considers co-clustering as a matrix approximation. The trifactorization approach is considered and algorithms based on update rules are described. Links with numerical and probabi, Englisch, Ebook.
Co-Clustering: Cluster or co-cluster analyses are important tools in a variety of scientific areas. The introduction of this book presents a state of the art of already well-established, as well as more recent methods of co-clustering. The authors mainly deal with the two-mode partitioning under different approaches, but pay particular attention to a probabilistic approach. Chapter 1 concerns clustering in general and the model-based clustering in particular. The authors briefly review the classical clustering methods and focus on the mixture model. They present and discuss the use of different mixtures adapted to different types of data. The algorithms used are described and related works with different classical methods are presented and commented upon. This chapter is useful in tackling the problem of co-clustering under the mixture approach. Chapter 2 is devoted to the latent block model proposed in the mixture approach context. The authors discuss this model in detail and present its interest regarding co-clustering. Various algorithms are presented in a general context. Chapter 3 focuses on binary and categorical data. It presents, in detail, the appropriated latent block mixture models. Variants of these models and algorithms are presented and illustrated using examples. Chapter 4 focuses on contingency data. Mutual information, phi-squared and model-based co-clustering are studied. Models, algorithms and connections among different approaches are described and illustrated. Chapter 5 presents the case of continuous data. In the same way, the different approaches used in the previous chapters are extended to this situation. Contents 1. Cluster Analysis. 2. Model-Based Co-Clustering. 3. Co-Clustering of Binary and Categorical Data. 4. Co-Clustering of Contingency Tables. 5. Co-Clustering of Continuous Data. About the Authors Gérard Govaert is Professor at the University of Technology of Compiègne, France. He is also a member of the CNRS Laboratory Heudiasyc (Heuristic and diagnostic of complex systems). His research interests include latent structure modeling, model selection, model-based cluster analysis, block clustering and statistical pattern recognition. He is one of the authors of the MIXMOD (MIXtureMODelling) software. Mohamed Nadif is Professor at the University of Paris-Descartes, France, where he is a member of LIPADE (Paris Descartes computer science laboratory) in the Mathematics and Computer Science department. His research interests include machine learning, data mining, model-based cluster analysis, co-clustering, factorization and data analysis. Cluster Analysis is an important tool in a variety of scientific areas. Chapter 1 briefly presents a state of the art of already well-established as well more recent methods. The hierarchical, partitioning and fuzzy approaches will be discussed amongst others. The authors review the difficulty of these classical methods in tackling the high dimensionality, sparsity and scalability. Chapter 2 discusses the interests of coclustering, presenting different approaches and defining a co-cluster. The authors focus on co-clustering as a simultaneous clustering and discuss the cases of binary, continuous and co-occurrence data. The criteria and algorithms are described and illustrated on simulated and real data. Chapter 3 considers co-clustering as a model-based co-clustering. A latent block model is defined for different kinds of data. The estimation of parameters and co-clustering is tackled under two approaches: maximum likelihood and classification maximum likelihood. Hard and soft algorithms are described and applied on simulated and real data. Chapter 4 considers co-clustering as a matrix approximation. The trifactorization approach is considered and algorithms based on update rules are described. Links with numerical and probabi, Englisch, Ebook.
4

Co-Clustering (2013)

ISBN: 9781118649497 bzw. 1118649494, in Englisch, Wiley John + Sons, neu, E-Book.
Lieferung aus: Schweiz, Sofort per Download lieferbar.
Models, Algorithms and Applications, Cluster or co-cluster analyses are important tools in a variety of scientific areas. The introduction of this book presents a state of the art of already well-established, as well as more recent methods of co-clustering. The authors mainly deal with the two-mode partitioning under different approaches, but pay particular attention to a probabilistic approach.Chapter 1 concerns clustering in general and the model-based clustering in particular. The authors briefly review the classical clustering methods and focus on the mixture model. They present and discuss the use of different mixtures adapted to different types of data. The algorithms used are described and related works with different classical methods are presented and commented upon. This chapter is useful in tackling the problem ofco-clustering under the mixture approach. Chapter 2 is devoted to the latent block model proposed in the mixture approach context. The authors discuss this model in detail and present its interest regarding co-clustering. Various algorithms are presented in a general context. Chapter 3 focuses on binary and categorical data. It presents, in detail, the appropriated latent block mixture models. Variants of these models and algorithms are presented and illustrated using examples. Chapter 4 focuses on contingency data. Mutual information, phi-squared and model-based co-clustering are studied. Models, algorithms and connections among different approaches are described and illustrated. Chapter 5 presents the case of continuous data. In the same way, the different approaches used in the previous chapters are extended to this situation.Contents1. Cluster Analysis.2. Model-Based Co-Clustering.3. Co-Clustering of Binary and Categorical Data.4. Co-Clustering of Contingency Tables.5. Co-Clustering of Continuous Data.About the AuthorsGérard Govaert is Professor at the University of Technology of Compiègne, France. He is also a member of the CNRS Laboratory Heudiasyc (Heuristic and diagnostic of complex systems). His research interests include latent structure modeling, model selection, model-based cluster analysis, block clustering and statistical pattern recognition. He is one of the authors of the MIXMOD (MIXtureMODelling) software.Mohamed Nadif is Professor at the University of Paris-Descartes, France, where he is a member of LIPADE (Paris Descartes computer science laboratory) in the Mathematics and Computer Science department. His research interests include machine learning, data mining, model-based cluster analysis, co-clustering, factorization and data analysis.Cluster Analysis is an important tool in a variety of scientific areas. Chapter 1 briefly presents a state of the art of already well-established as well more recent methods. The hierarchical, partitioning and fuzzy approaches will be discussed amongst others. The authors review the difficulty of these classical methods in tackling the high dimensionality, sparsity and scalability. Chapter 2 discusses the interests of coclustering, presenting different approaches and defining a co-cluster. The authors focus on co-clustering as a simultaneous clustering and discuss the cases of binary, continuous and co-occurrence data. The criteria and algorithms are described and illustrated on simulated and real data. Chapter 3 considers co-clustering as a model-based co-clustering. A latent block model is defined for different kinds of data. The estimation of parameters and co-clustering is tackled under two approaches: maximum likelihood and classification maximum likelihood. Hard and soft algorithms are described and applied on simulated and real data. Chapter 4 considers co-clustering as a matrix approximation. The trifactorization approach is considered and algorithms based on update rules are described. Links with numerical and probabilistic approaches are established. A combination of algorithms are proposed and evaluated on simulated and real data. Chapter 5 considers a co-clustering or bi-clustering as the search for coherent co-clusters in biological terms or the extraction of co-clusters under conditions. Classical algorithms will be described and evaluated on simulated and real data. Different indices to evaluate the quality of coclusters are noted and used in numerical experiments. PDF, 11.12.2013.
Models, Algorithms and Applications, Cluster or co-cluster analyses are important tools in a variety of scientific areas. The introduction of this book presents a state of the art of already well-established, as well as more recent methods of co-clustering. The authors mainly deal with the two-mode partitioning under different approaches, but pay particular attention to a probabilistic approach.Chapter 1 concerns clustering in general and the model-based clustering in particular. The authors briefly review the classical clustering methods and focus on the mixture model. They present and discuss the use of different mixtures adapted to different types of data. The algorithms used are described and related works with different classical methods are presented and commented upon. This chapter is useful in tackling the problem ofco-clustering under the mixture approach. Chapter 2 is devoted to the latent block model proposed in the mixture approach context. The authors discuss this model in detail and present its interest regarding co-clustering. Various algorithms are presented in a general context. Chapter 3 focuses on binary and categorical data. It presents, in detail, the appropriated latent block mixture models. Variants of these models and algorithms are presented and illustrated using examples. Chapter 4 focuses on contingency data. Mutual information, phi-squared and model-based co-clustering are studied. Models, algorithms and connections among different approaches are described and illustrated. Chapter 5 presents the case of continuous data. In the same way, the different approaches used in the previous chapters are extended to this situation.Contents1. Cluster Analysis.2. Model-Based Co-Clustering.3. Co-Clustering of Binary and Categorical Data.4. Co-Clustering of Contingency Tables.5. Co-Clustering of Continuous Data.About the AuthorsGérard Govaert is Professor at the University of Technology of Compiègne, France. He is also a member of the CNRS Laboratory Heudiasyc (Heuristic and diagnostic of complex systems). His research interests include latent structure modeling, model selection, model-based cluster analysis, block clustering and statistical pattern recognition. He is one of the authors of the MIXMOD (MIXtureMODelling) software.Mohamed Nadif is Professor at the University of Paris-Descartes, France, where he is a member of LIPADE (Paris Descartes computer science laboratory) in the Mathematics and Computer Science department. His research interests include machine learning, data mining, model-based cluster analysis, co-clustering, factorization and data analysis.Cluster Analysis is an important tool in a variety of scientific areas. Chapter 1 briefly presents a state of the art of already well-established as well more recent methods. The hierarchical, partitioning and fuzzy approaches will be discussed amongst others. The authors review the difficulty of these classical methods in tackling the high dimensionality, sparsity and scalability. Chapter 2 discusses the interests of coclustering, presenting different approaches and defining a co-cluster. The authors focus on co-clustering as a simultaneous clustering and discuss the cases of binary, continuous and co-occurrence data. The criteria and algorithms are described and illustrated on simulated and real data. Chapter 3 considers co-clustering as a model-based co-clustering. A latent block model is defined for different kinds of data. The estimation of parameters and co-clustering is tackled under two approaches: maximum likelihood and classification maximum likelihood. Hard and soft algorithms are described and applied on simulated and real data. Chapter 4 considers co-clustering as a matrix approximation. The trifactorization approach is considered and algorithms based on update rules are described. Links with numerical and probabilistic approaches are established. A combination of algorithms are proposed and evaluated on simulated and real data. Chapter 5 considers a co-clustering or bi-clustering as the search for coherent co-clusters in biological terms or the extraction of co-clusters under conditions. Classical algorithms will be described and evaluated on simulated and real data. Different indices to evaluate the quality of coclusters are noted and used in numerical experiments. PDF, 11.12.2013.
5

Co-Clustering (2013)

ISBN: 9781118649497 bzw. 1118649494, in Englisch, Wiley John + Sons, neu, E-Book.
Models, Algorithms and Applications Cluster or co-cluster analyses are important tools in a variety of scientific areas. The introduction of this book presents a state of the art of already well-established, as well as more recent methods of co-clustering. The authors mainly deal with the two-mode partitioning under different approaches, but pay particular attention to a probabilistic approach.Chapter 1 concerns clustering in general and the model-based clustering in particular. The authors briefly review the classical clustering methods and focus on the mixture model. They present and discuss the use of different mixtures adapted to different types of data. The algorithms used are described and related works with different classical methods are presented and commented upon. This chapter is useful in tackling the problem ofco-clustering under the mixture approach. Chapter 2 is devoted to the latent block model proposed in the mixture approach context. The authors discuss this model in detail and present its interest regarding co-clustering. Various algorithms are presented in a general context. Chapter 3 focuses on binary and categorical data. It presents, in detail, the appropriated latent block mixture models. Variants of these models and algorithms are presented and illustrated using examples. Chapter 4 focuses on contingency data. Mutual information, phi-squared and model-based co-clustering are studied. Models, algorithms and connections among different approaches are described and illustrated. Chapter 5 presents the case of continuous data. In the same way, the different approaches used in the previous chapters are extended to this situation.Contents1. Cluster Analysis.2. Model-Based Co-Clustering.3. Co-Clustering of Binary and Categorical Data.4. Co-Clustering of Contingency Tables.5. Co-Clustering of Continuous Data.About the AuthorsGérard Govaert is Professor at the University of Technology of Compiègne, France. He is also a member of the CNRS Laboratory Heudiasyc (Heuristic and diagnostic of complex systems). His research interests include latent structure modeling, model selection, model-based cluster analysis, block clustering and statistical pattern recognition. He is one of the authors of the MIXMOD (MIXtureMODelling) software.Mohamed Nadif is Professor at the University of Paris-Descartes, France, where he is a member of LIPADE (Paris Descartes computer science laboratory) in the Mathematics and Computer Science department. His research interests include machine learning, data mining, model-based cluster analysis, co-clustering, factorization and data analysis.Cluster Analysis is an important tool in a variety of scientific areas. Chapter 1 briefly presents a state of the art of already well-established as well more recent methods. The hierarchical, partitioning and fuzzy approaches will be discussed amongst others. The authors review the difficulty of these classical methods in tackling the high dimensionality, sparsity and scalability. Chapter 2 discusses the interests of coclustering, presenting different approaches and defining a co-cluster. The authors focus on co-clustering as a simultaneous clustering and discuss the cases of binary, continuous and co-occurrence data. The criteria and algorithms are described and illustrated on simulated and real data. Chapter 3 considers co-clustering as a model-based co-clustering. A latent block model is defined for different kinds of data. The estimation of parameters and co-clustering is tackled under two approaches: maximum likelihood and classification maximum likelihood. Hard and soft algorithms are described and applied on simulated and real data. Chapter 4 considers co-clustering as a matrix approximation. The trifactorization approach is considered and algorithms based on update rules are described. Links with numerical and probabilistic approaches are established. A combination of algorithms are proposed and evaluated on simulated and real data. Chapter 5 considers a co-clustering or bi-clustering as the search for coherent co-clusters in biological terms or the extraction of co-clusters under conditions. Classical algorithms will be described and evaluated on simulated and real data. Different indices to evaluate the quality of coclusters are noted and used in numerical experiments. 11.12.2013, PDF.
6
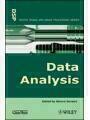
Data Analysis

ISBN: 9780470610312 bzw. 047061031X, in Deutsch, Wiley, Vereinigte Staaten von Amerika, neu, E-Book.
Lieferung aus: Deutschland, zzgl. Versandkosten, Sofort per Download lieferbar.
Data Analysis, The first part of this book is devoted to methods seeking relevant dimensions of data. The variables thus obtained provide a synthetic description which often results in a graphical representation of the data. After a general presentation of the discriminating analysis, the second part is devoted to clustering methods which constitute another method, often complementary to the methods described in the first part, to synthesize and to analyze the data. The book concludes by examining the links existing between data mining and data analysis.
Data Analysis, The first part of this book is devoted to methods seeking relevant dimensions of data. The variables thus obtained provide a synthetic description which often results in a graphical representation of the data. After a general presentation of the discriminating analysis, the second part is devoted to clustering methods which constitute another method, often complementary to the methods described in the first part, to synthesize and to analyze the data. The book concludes by examining the links existing between data mining and data analysis.
Lade…